Table of Content
05 March 2018, Volume 69 Issue 3
Office Online
For Authors
For Reviewers
Journal Online
Information
-
CN 11-1946/TQ
ISSN 0438-1157 -
Superintended:
China Association for Science and Technology -
Sponsored:
Chemical Industry and Engineering
Society of China,
Chemical Industry Press Co., Ltd. -
Published:
Chemical Industry Press Co., Ltd. -
Editor in Chief:
LI Jinghai -
Domestic distributed:
Local post office
Distribution code:2-370 -
Abroad distributed:
China International Book Trading
Corporation
Distribution code:M6081 -
Editorial office:
No.13 Qingnianhu South Street,
Dongcheng District,
Beijing 100011,China
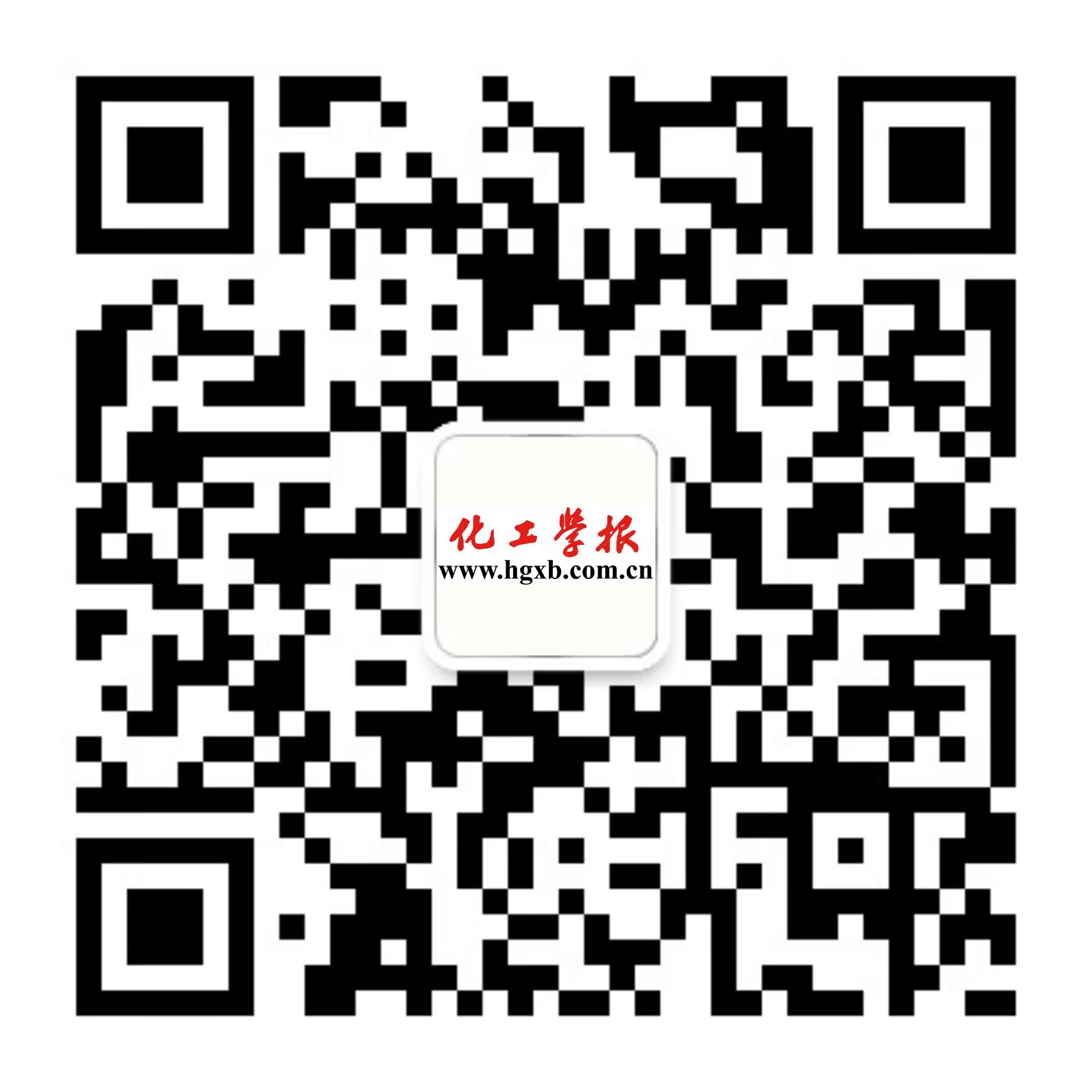
CIESC Journal Wechat